Enhancing Science Journalism with Collaborative LLMs: A New Approach to Accessible Writing
In today's rapidly advancing world, keeping the general public informed about the latest scientific discoveries is crucial, yet the technical nature of most scientific writing poses a significant barrier. Science journalism, traditionally tasked with translating complex research into accessible articles, faces the challenge of making highly specialized content understandable to a broad audience.
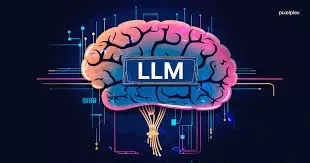
This task becomes even more difficult as the sheer volume of scientific knowledge continues to grow. While automation could help ease the burden of generating science-related content, traditional methods—such as fine-tuning models on small datasets—often fall short when it comes to readability. Enter the concept of Automatic Science Journalism (ASJ), which leverages large language models (LLMs) to improve this process by generating accessible science news.
In this study, they proposes the JRE-L framework, an innovative approach to ASJ that integrates three LLMs working collaboratively. The framework involves iterative cycles of writing, reading, feedback, and revision to produce science articles that are not only accurate but also highly readable. The results suggest that this collaboration is a step forward in creating accessible content for the general public.
The Challenge of Science Journalism
The primary goal of science journalism is to make complex, technical content digestible for readers who lack the necessary domain expertise. Current scientific literature, including press releases and academic papers, is often tailored for researchers and scientists. This makes it challenging for the general public to grasp key concepts without assistance.
While some progress has been made with parallel corpora and summarization techniques, these approaches often produce content that remains too technical or difficult for the general public to comprehend. With the growing role of LLMs in natural language processing (NLP), however, there's potential to take science journalism a step further by using these models to facilitate more effective communication.
JRE-L Framework: Collaborative LLMs for Better Readability
The JRE-L framework takes a novel approach to science communication by simulating the writing-feedback-revision process. This model relies on three distinct LLMs:
-
The Journalist: The first LLM takes on the role of the journalist, generating an article based on a scientific paper. This model is tasked with converting complex research findings into accessible language.
-
The Reader: A second, smaller LLM acts as the reader, providing feedback on the article from the perspective of someone who lacks deep domain knowledge. This feedback focuses on identifying parts of the article that are difficult to understand or unclear to a general audience.
-
The Editor: The third LLM evaluates the reader’s feedback and suggests revisions to the journalist's article, helping to improve its clarity, readability, and accuracy.
By simulating a human-driven feedback loop, the JRE-L framework allows for iterative refinement, progressively making the article more accessible with each cycle. This collaborative process is inspired by real-world journalism, where journalists often revise their work based on editor and reader feedback.
Empirical Results: Higher Readability, Same Accuracy
To evaluate the effectiveness of the JRE-L framework, we conducted extensive experiments comparing it with existing methods, including single LLM-based approaches and other LLM collaboration strategies. Our findings demonstrate that the JRE-L framework outperforms these alternatives in terms of readability, producing content that is significantly easier for general readers to understand.
In addition to improving readability, the articles generated through the JRE-L process maintain competitive levels of technical accuracy. This balance of accessibility and correctness is crucial for science journalism, where precision is just as important as clarity.
Understanding the Iterative Process: Improving Through Feedback
The iterative nature of the JRE-L framework is key to its success. Each cycle of feedback and revision serves to improve the article incrementally. By utilizing the reader's feedback, the journalist can address areas that may be overly technical, unclear, or difficult for non-experts to follow. The editor, in turn, ensures that the final article retains its accuracy while also becoming more readable.
This iterative refinement is akin to how human writers, editors, and readers collaborate in traditional journalism. The use of LLMs in this process, however, accelerates the cycle, allowing for rapid improvements in writing and ensuring that articles are consistently accessible.
Implications and Future Directions
The JRE-L framework represents a significant step forward in using LLMs for Automatic Science Journalism. By addressing the challenges of readability and accessibility, this framework could become an essential tool for science communicators in the future. Furthermore, the collaborative nature of the LLMs in the JRE-L framework offers a new perspective on how AI can enhance human creativity and communication.
Looking ahead, there are several directions for future research. One possibility is to further refine the interaction between the three LLMs to allow for more personalized adjustments based on the audience's knowledge level. Additionally, exploring the integration of multimodal content (such as images or videos) could provide more engaging and informative science journalism.
Conclusion: A Step Forward in Science Communication
The JRE-L framework introduces a powerful new way to use LLMs to bridge the gap between complex scientific knowledge and the general public. By iterating through cycles of writing, reading, feedback, and revision, this framework ensures that science articles are both technically accurate and highly readable. As the need for accessible science journalism continues to grow, the JRE-L framework represents an exciting development in the field of automatic science communication.How do you think AI tools like LLMs can continue to improve the accessibility of scientific articles for non-experts? What are the challenges and opportunities in integrating AI into traditional science journalism? Feel free to share your thoughts in the comments!
What's Your Reaction?
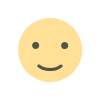
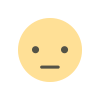
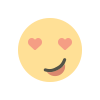
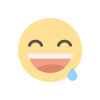
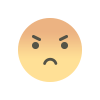
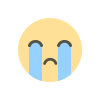
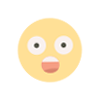