A Modern Conversation on Language, Machines, and Linguistics: Norm, Claudette, and the Rise of Language Models
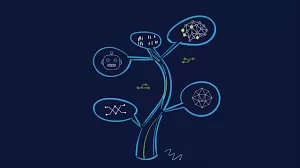
It’s 1968, and Norm is discussing his theory on human languages with Claudette during lunch. Norm argues that all human languages share a deep, underlying structure—an intricate system of rules and principles that govern surface forms. Claudette, on the other hand, is captivated by the idea of machines that can understand and speak human languages, dreaming of building a machine that could converse fluently in any language. The conversation touches on the possibility of applying Norm’s linguistic theories to build such a system, but they remain divided in their focus: Norm is interested in understanding the mind and human language, while Claudette is excited by engineering solutions that make machines talk. Little do they know, these two ideas will converge decades later with the advent of language models.
Fast forward to 2025: Claudette’s dream of having machines that can talk and understand language has come true. Language models now exhibit impressive fluency in human languages, often producing grammatically correct and sensible sentences. But does this success challenge the linguistic theories Norm spent his career developing? Is there a fundamental connection between these machines and the deep structures Norm studied? Or are these machines, despite their apparent linguistic competence, simply performing statistical tasks that don’t reflect the true nature of human language?
Norm's Concerns: Are Language Models Missing the Point?
Norm might initially dismiss these language models, given their reliance on data-driven statistical methods rather than formal rules of linguistic structure. He could argue that, unlike the human brain or traditional linguistic models, language models like those Claudette envisioned are not grounded in any true understanding of language. They operate based on statistical associations learned from vast amounts of data, without necessarily capturing the deeper, more abstract principles that linguists have spent years studying.
This skepticism is rooted in the belief that neural models, while effective at pattern recognition, cannot offer any true insights into the cognitive structures that underlie human language. They may generate sentences that appear grammatically correct but might not truly understand the mechanisms at play. After all, if these models can be trained on anything, what does it really tell us about language? Are they just “mathematical clay,” capable of mimicking language without any deep understanding?
Norm’s perspective might suggest that understanding the brain and the true nature of language requires more than just a collection of observed patterns—it needs a deeper theory of how language operates cognitively and structurally. The complexity of human thought and language cannot be reduced to a series of statistical approximations, he might argue. After all, what use are systems that "speak" if they don't truly capture the essence of language?
Claudette's Perspective: Practical Success, Regardless of the Method
On the other hand, Claudette, who has built these language models and witnessed their real-world success, might be less concerned with how these machines work at a theoretical level. She has what she always wanted—a machine that can talk and understand. The results are tangible, and the applications are impressive. For her, the goal was never to build a perfect model of human language, but to create something that could engage with people, solve problems, and communicate effectively.
The beauty of this approach lies in its simplicity: Claudette doesn’t need to worry about the specifics of how the machine understands language, as long as it works. And in practical terms, these models do—they’ve been trained on vast datasets, and they generate linguistically competent text. For Claudette, this is an engineering triumph that bypasses theoretical concerns and brings language technology to fruition.
Moreover, Claudette’s perspective could lead to a more pragmatic view of language. The focus on statistical modeling doesn’t require adherence to any one theory of linguistic structure. The approach can evolve, learning from massive amounts of real-world data and adapting to the complexity of human language in all its forms.
The Middle Ground: What Language Models Teach Us About Language
However, as we move into the current landscape, a new perspective seems to be gaining traction—a middle ground between Norm's theoretical linguistics and Claudette’s engineering-driven success. Language models, despite their reliance on statistical methods, do reveal important insights into how language works. In fact, these models have been shown to learn structural patterns in language that are not only non-trivial but deeply informative.
Language models like GPT-4 and ChatGPT have demonstrated remarkable capabilities in understanding and generating text that follows syntactic and semantic structures, even capturing dependencies that are core to linguistic theory, such as subject-verb agreement and hierarchical relationships within sentences. These models have been trained on enormous amounts of text data, learning patterns and associations that allow them to generalize and generate coherent text. Their performance on tasks like grammar correction, machine translation, and question answering suggest that they are, in fact, capturing aspects of language structure, even if their internal representations differ from the formal symbolic theories Norm proposed.
The key insight here is that these models, despite not being based on any specific theory of language, may still provide valuable evidence about the nature of linguistic competence. The fact that they are capable of performing complex language tasks suggests that there is something about language—whether statistical, cognitive, or structural—that they are tapping into. By studying the way language models generate and understand language, researchers may gain new insights into how the human mind processes language and how language itself can be modeled computationally.
This middle ground also provides a more holistic view of language science. Rather than dismissing neural models as irrelevant or traditional linguistic theories as outdated, we can recognize that both approaches have something to offer. Language models do not replace the need for linguistic theory—they complement it, offering a new way of thinking about how language functions and how it can be learned and processed.
Conclusion: Bridging the Gap Between Theory and Practice
As Norm and Claudette sit down for their long-awaited lunch in 2025, their conversation will likely be one of mutual curiosity and excitement. Both have come a long way, but their ideas are far from incompatible. The success of Claudette’s language models does not invalidate Norm’s theories of language; rather, it provides a new lens through which to explore the very questions that have fascinated linguists for centuries. Language models, though different in approach, offer valuable insights into language structure, learning, and processing that can inform linguistic theory and cognitive science.
At the same time, linguistic theory has much to offer in terms of understanding the deep structures that underpin language models. By combining insights from both fields, we can move toward a more comprehensive understanding of language—one that integrates theoretical, computational, and empirical approaches. As Norm and Claudette reconnect, they will likely find that their separate paths have led them to a shared destination: the realization that language, in all its complexity, can be understood and modeled in ways that neither could have imagined alone.
What's Your Reaction?
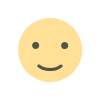
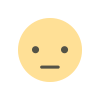
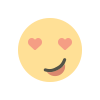
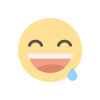
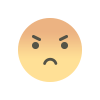
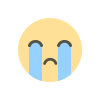
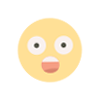