Enhancing Irony Detection with ChatGPT: A Novel Approach to Contextual Understanding and Reasoning
The Challenge of Irony in NLP: Irony is a complex and powerful form of figurative language (FL) that often introduces subtle meanings, making it difficult for natural language processing (NLP) systems to detect and interpret. In recent years, irony has become increasingly prevalent on social media platforms, complicating tasks like sentiment analysis, recommendation systems, and misinformation detection. Understanding irony is crucial to mitigate its negative impacts, as misinterpretation can lead to incorrect conclusions in NLP applications.
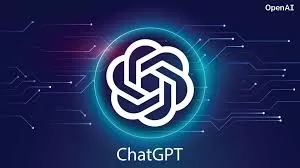
Irony can take many forms, such as verbal irony—where a speaker says something but means the opposite—or situational irony, where the outcome of a situation is the opposite of what was expected. This nuanced nature of irony, along with its reliance on context, tone, and cultural references, makes it a significant challenge for current AI models to detect. While large language models (LLMs) like ChatGPT have shown remarkable progress in capturing contextual and implicit meanings, irony detection remains an area where further innovation is needed. In this study, we explore how ChatGPT's zero-shot learning capabilities can be enhanced for irony detection through a novel prompt engineering framework—IDADP (Irony Detection and Reasoning Prompt)—to improve its generalization, reasoning, and understanding of irony across diverse datasets.
Understanding the Core Concepts
-
Irony in Language: Irony involves expressing one’s meaning using language that normally signifies the opposite. It's commonly used to convey humor, sarcasm, or a form of emphasis. Detecting irony requires an understanding of both the literal meaning and the underlying intent.
-
Zero-shot Learning: Zero-shot learning allows models to make predictions on tasks they were not explicitly trained for by using prior knowledge and generalization capabilities. In the case of irony detection, a zero-shot model like ChatGPT can potentially identify and understand ironic statements across various datasets without being trained on specific examples.
-
Prompt Engineering: Prompt engineering involves crafting specific inputs that guide LLMs like ChatGPT to perform tasks more effectively. In this study, we use prompt engineering to enhance the model’s ability to understand and reason about irony, leading to more accurate detection.
The IDADP Framework: Revolutionizing Irony Detection
The IDADP, a framework designed to improve ChatGPT's zero-shot performance on irony detection. The framework consists of several key components, each aimed at addressing the limitations in existing models:
1. Domain-Specific Knowledge Integration
By leveraging domain-specific knowledge, we can guide ChatGPT to better understand the context in which irony occurs. This knowledge is incorporated into the model through carefully designed prompts that specify the context, tone, and possible ironic expressions relevant to the domain. This step ensures that ChatGPT is better equipped to understand subtle cues, such as sarcasm or exaggerated statements, that are central to detecting irony.
2. Prompt Engineering for Reasoning
Effective irony detection requires reasoning—especially when dealing with indirect or subtle forms of irony. The IDADP framework incorporates reasoning-driven prompts that allow ChatGPT to make logical inferences about the intent behind a statement. By guiding the model to consider not just the words, but also the broader context and implied meaning, we improve its ability to detect and explain irony accurately.
3. Majority Voting for Enhanced Generalization
Given the variety of ironic expressions across different datasets, we employ a majority voting ensemble to combine the predictions from multiple outputs. This approach helps ensure that the model’s final classification is robust and generalizes well to unseen scenarios. The ensemble method strengthens the model's ability to accurately detect irony in various forms, whether it's verbal or situational irony, across different social media platforms.
Experimental Setup and Datasets
We evaluate the performance of the IDADP framework across six irony detection datasets that span different genres, including social media conversations, product reviews, and news articles. These datasets include:
- Sarcasm Detection on Reddit
- Irony in Tweets
- Humor and Sarcasm in Online Reviews
- Situational Irony in News Articles
- Sarcasm Detection in Product Reviews
- Irony in Political Speech
Each dataset contains both ironic and non-ironic statements, and we focus on improving the model's ability to generalize across these different types of irony.
Key Findings and Contributions
Our experiments demonstrate that ChatGPT, when combined with the IDADP framework, significantly outperforms other state-of-the-art zero-shot models in irony detection. The following contributions highlight the impact of our approach:
-
Improved Accuracy: The IDADP framework boosts irony detection accuracy by addressing issues of generalization and reasoning, which are often problematic in existing models. ChatGPT with IDADP achieved a significant improvement in identifying both verbal and situational irony across diverse datasets.
-
Enhanced Understanding and Reasoning: The combination of domain-specific prompts and reasoning-driven design allows the model to generate more accurate and coherent explanations for why a statement is considered ironic, improving transparency and trustworthiness in the system.
-
Increased Robustness: The majority voting mechanism further enhances the system’s ability to handle diverse forms of irony, improving its generalization ability across unseen data and real-world scenarios.
Challenges and Future Directions
While the IDADP framework offers promising results, several challenges remain:
-
Cultural and Contextual Differences: Irony can vary significantly across cultures, contexts, and platforms. Future work will explore how to adapt the model to different cultural references and language use.
-
Multimodal Irony Detection: To further improve the system’s understanding of irony, integrating multimodal data, such as images or videos alongside text, could provide more contextual cues to enhance detection.
-
Hybrid Symbolic-Neural Models: Combining symbolic reasoning with neural models could further improve the model’s ability to detect irony by incorporating explicit knowledge about irony and sarcasm.
Conclusion: A Step Forward in Irony Detection
The IDADP framework, which significantly enhances ChatGPT's zero-shot capabilities for irony detection. By carefully crafting domain-specific prompts and focusing on reasoning, that demonstrated improved performance across multiple datasets. Our findings indicate that irony detection, particularly in real-world scenarios, can benefit greatly from contextual understanding, reasoning, and transparency in model decision-making. Moving forward, this work sets the stage for further advancements in irony detection and understanding, offering a pathway for more reliable and robust NLP systems.What do you think could be the next frontier in enhancing models for irony detection? Could integrating multimodal data, such as images or videos, play a key role in improving understanding, or is there another area where we should focus our attention for even greater accuracy?
What's Your Reaction?
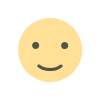
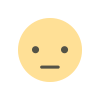
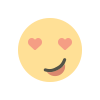
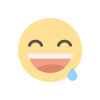
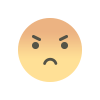
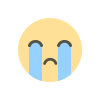
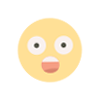