3D-MoE: Revolutionizing 3D Vision with Multi-Modal Expertise
In a world that is inherently three-dimensional, the pursuit of technology that can interpret and interact with this space just as humans do remains a significant frontier in computer science. 3D vision, an essential facet of this endeavor, seeks to improve how machines perceive and understand spatial environments. Historically, the field has leaned heavily on 2D data, which is plentiful but lacks the depth necessary for numerous complex tasks. Recent advancements, however, have ushered in a new era of 3D vision technologies, particularly through the integration of sophisticated large language models (LLMs).
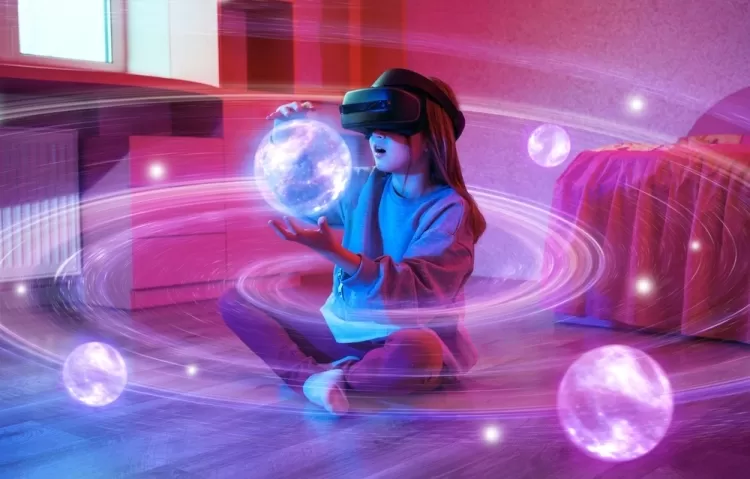
Understanding the Core Concepts
Key Terminology: At the heart of this discussion are Large Language Models (LLMs), which process vast amounts of text to understand and generate human-like text. In the realm of 3D vision, these models are becoming increasingly multimodal, meaning they can interpret and synthesize information from various types of data, including visual, textual, and spatial inputs.
Background Information: The traditional approach to computer vision predominantly utilizes 2D images which are abundant and straightforward to process but fall short in tasks requiring depth perception. The transition towards 3D models allows for more accurate spatial reasoning, crucial for advanced applications like robotic navigation and complex scene understanding.
The Challenge Addressed by the Research
Current Limitations: Traditional 2D-focused models are often inadequate for tasks that need an understanding of the depth and spatial relationships between objects in a scene. This limitation is a significant hurdle in applications ranging from autonomous driving to augmented reality.
Real-World Implications: The inability to effectively parse 3D environments can lead to inefficiencies and errors in applications that require high degrees of accuracy, such as surgical robotics and automated warehousing.
A New Approach
Innovative Solution: The research introduces a new model, 3D-MoE (3D Mixture-of-Experts), which integrates mixture-of-experts techniques with multimodal LLMs to enhance 3D vision capabilities. This model leverages existing knowledge from pretrained LLMs while optimizing them through a novel architecture designed specifically for 3D data.
How It Works: By partitioning the model into different 'expert' components, each specializing in a specific type of data input, 3D-MoE efficiently processes and integrates diverse data types. This structure allows the model to use fewer resources during inference, making it both faster and more scalable.
Key Findings: The integration of a diffusion-based module, known as Pose-DiT, enables the model to predict the 6D pose of objects, facilitating more nuanced interactions with 3D environments. Tests show that this approach not only reduces computational demands but also significantly improves performance in 3D task scenarios.
Implications for the Field
Practical Applications: The enhanced ability to understand and interact with 3D spaces could revolutionize several industries, including robotics, virtual reality, and autonomous vehicle navigation, where precise spatial awareness is paramount.
Future Research: The framework opens avenues for further exploration into more efficient training methods for multimodal models and their application across different types of 3D data.
Conclusion: A Step Forward in Computer Vision and AI
Summary: The development of 3D-MoE marks a significant advancement in the field of computer vision, addressing many of the traditional limitations faced by 2D models with a novel and effective solution.
Broader Impact: By improving how machines perceive and interact with three-dimensional spaces, this research could have far-reaching effects on technology development, impacting everything from healthcare to entertainment.
Final Thoughts: As we continue to push the boundaries of what artificial intelligence can achieve, the evolution of models like 3D-MoE highlights the incredible potential of integrating different types of intelligence to better understand and interact with our world.
Engage With Us
How do you envision the future of 3D technologies in everyday life? Could models like 3D-MoE transform your professional field or personal experiences? Join the conversation below!
What's Your Reaction?
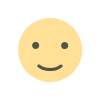
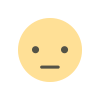
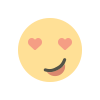
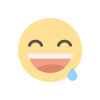
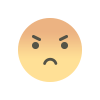
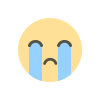
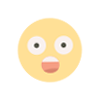