Evaluating Multimodal Large Language Models for Industrial Applications: The MME-Industry Benchmark
In the rapidly advancing field of artificial intelligence (AI), Multimodal Large Language Models (MLLMs) have become central to solving complex real-world problems. These models are designed to process and generate information across multiple modalities, such as text, images, and videos, making them highly effective for diverse tasks in industries like healthcare, finance, and manufacturing. However, as these models become increasingly integrated into industrial applications, their real-world efficacy across different sectors remains underexplored.
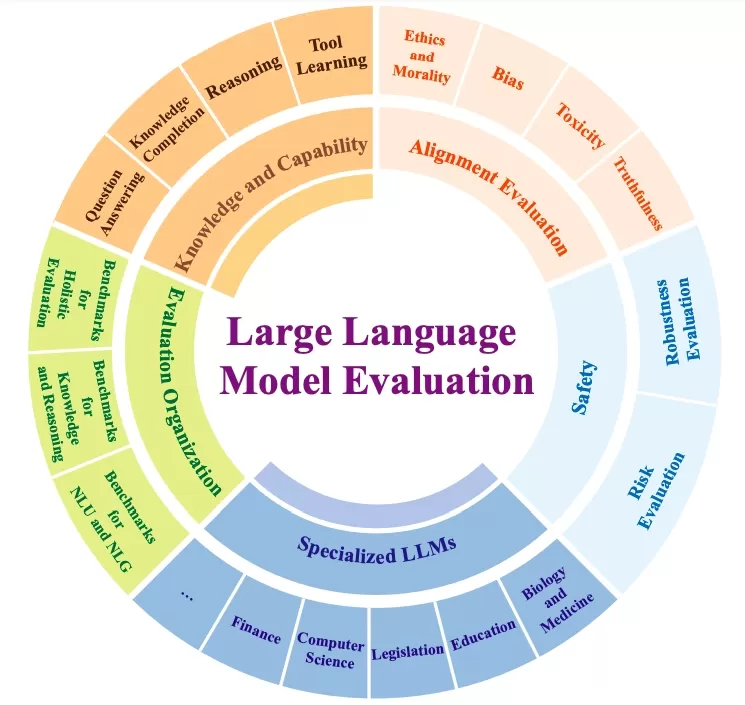
While we have seen numerous evaluation benchmarks for MLLMs, such as those focused on document understanding or text recognition, comprehensive assessments that address industrial use cases are still scarce. A key challenge is the need for evaluation tools that can test MLLMs across a broad spectrum of industries and ensure that their performance is both accurate and relevant to real-world problems.
Problem Statement:
There is a notable gap in existing benchmarks for evaluating MLLMs in the context of industrial applications. While models like GPT-4 and various vision-language models have made strides in capabilities, there are few tools that specifically assess their ability to handle the nuances and specialized knowledge required in industrial settings. Given the variety of sectors within industry—each with its own complexities—the need for a robust evaluation benchmark that spans different verticals is critical.
Research Overview:
To fill this gap, a new benchmark called MME-Industry has been introduced. This benchmark is designed specifically to evaluate MLLMs in industrial environments, covering 21 distinct sectors ranging from power generation to automotive manufacturing. It comprises 1,050 high-resolution images, each paired with a question and multiple-choice options, making it a comprehensive tool to test MLLMs’ capabilities in handling industry-specific queries. The dataset also includes both English and Chinese versions, allowing for cross-lingual evaluations.
Understanding the Core Concepts:
Key Terminology:
- Multimodal Large Language Models (MLLMs): These are AI models designed to understand and generate responses that involve multiple types of data inputs, such as images and text. For example, they can analyze an image and generate a relevant textual description or answer a question about it.
- Benchmarking: In the AI world, a benchmark is a standardized set of tasks or datasets used to evaluate the performance of models. Benchmarks help researchers assess how well models perform across different applications.
- Cross-lingual Evaluation: This involves testing models on tasks in multiple languages to understand their effectiveness beyond just one linguistic context.
Background Information:
MLLMs have evolved significantly, from early models like CLIP, which aligned images and text through contrastive learning, to more recent models like Flamingo and BLIP-2. However, the evaluation of these models has often been limited to generic tasks. To understand their industrial potential, the MME-Industry benchmark provides a structured environment to evaluate their performance in specific, real-world applications.
The Challenge Addressed by the Research:
Current Limitations:
Existing benchmarks for multimodal models often focus on specific tasks, such as image captioning, document understanding, or basic question answering. While these tasks are useful, they do not cover the depth and complexity found in industrial applications. Industrial environments require models to not only understand general visual and textual information but also to apply domain-specific knowledge to solve practical problems. This creates a significant challenge for MLLMs, as they must be able to navigate highly specialized contexts.
Real-World Implications:
In industries like power generation, electronics, and chemicals, the consequences of a model's failure to understand the intricacies of its domain can be severe. For example, incorrect identification of equipment in a factory or misunderstanding a safety procedure could lead to costly mistakes or even accidents. Therefore, it is critical to develop models that not only understand generic tasks but can also handle the complex, nuanced needs of industrial settings.
A New Approach:
Innovative Solution:
The MME-Industry benchmark offers a comprehensive approach by testing models across 21 distinct industrial sectors. This dataset includes images that require domain-specific knowledge to interpret correctly, ranging from questions about machinery in the power sector to queries about chemical safety protocols. The incorporation of non-OCR (Optical Character Recognition) questions ensures that models cannot rely solely on text recognition, pushing them to engage with the content in a more complex way.
How It Works:
Each of the 1,050 images is paired with a multiple-choice question and five possible answers, with only one correct response. This format encourages models to interpret the image content accurately and apply their understanding of industrial knowledge. Importantly, each sector’s questions are validated by industry experts, ensuring that the benchmark is grounded in real-world practices and expertise.
Key Findings:
Early experiments with state-of-the-art MLLMs have shown varying levels of success. Models like Qwen2-VL-72B-Instruct demonstrated superior performance across both English and Chinese evaluations, achieving 78.66% accuracy for Chinese and 75.04% for English. In contrast, models like MiniCPM-V-2.6 showed significant challenges, particularly in the Chinese industrial context. These findings underline the importance of evaluating MLLMs in both multilingual and industry-specific contexts.
Implications for the Field:
Practical Applications:
The MME-Industry benchmark opens new possibilities for MLLMs in industrial applications. With robust evaluation methods in place, industries can begin to assess which models are most effective for specific tasks. For example, a model that performs well in the electronics sector could be deployed for tasks like diagnosing faults in circuit boards, while one with strong performance in the chemical sector could help in safety protocol analysis or equipment maintenance.
Moreover, the availability of a multilingual dataset allows researchers to examine how well models generalize across languages. This could lead to models that are not only useful in global contexts but are also tailored to the needs of local industries.
Future Research:
The success of the MME-Industry benchmark suggests several directions for future research. First, the benchmark could be expanded to include more industrial sectors, particularly those that are emerging or underrepresented. Furthermore, as models continue to evolve, it would be valuable to test their ability to handle even more complex, real-world scenarios, such as real-time decision-making or multi-modal task execution (e.g., combining image and text analysis with real-time sensor data).
Conclusion: A Step Forward in Industrial AI:
Summary:
The introduction of MME-Industry represents a significant step forward in the evaluation of MLLMs for industrial applications. By providing a standardized and rigorous benchmark that spans 21 diverse sectors, it offers valuable insights into the capabilities of modern AI models in real-world contexts.
Broader Impact:
The implications of this research are far-reaching. As industries increasingly rely on AI for tasks ranging from quality control to safety monitoring, the ability to evaluate and fine-tune models for specific applications will be essential. MME-Industry offers a way to assess these capabilities, ensuring that AI models are not only innovative but also reliable and practical.
Final Thoughts:
As the field of industrial AI continues to grow, benchmarks like MME-Industry will be crucial in guiding future advancements. This benchmark not only highlights the current strengths and weaknesses of multimodal models but also sets the stage for more targeted research that could revolutionize industries worldwide.
How do you think the expansion of specialized evaluation benchmarks will impact the development of AI models for specific industries?
What's Your Reaction?
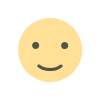
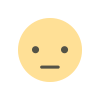
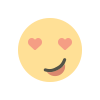
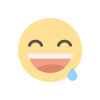
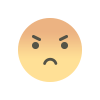
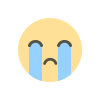
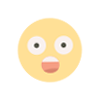