Through the Prism of Culture: Understanding the Nuances of Indian Subcultures with Large Language Models
In a world that is increasingly driven by artificial intelligence, one of the most profound challenges is ensuring that AI systems, particularly Large Language Models (LLMs), understand and respect the diversity of cultures and subcultures. In recent years, LLMs like GPT-4, Llama, and Gemini have demonstrated impressive language capabilities, but can they truly grasp the depth of cultural intricacies, especially when it comes to localized practices and traditions?
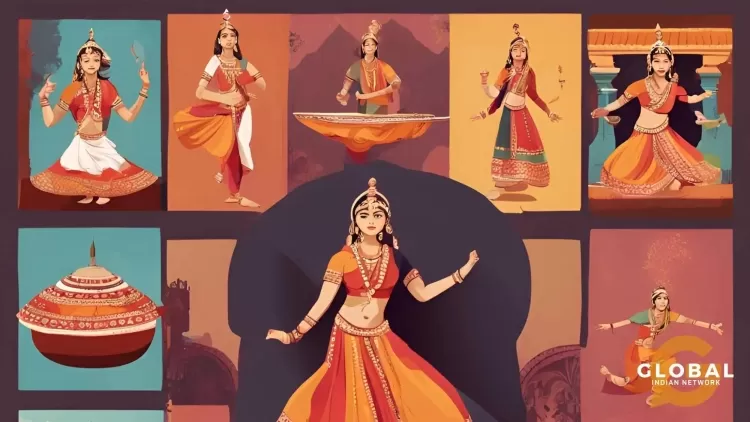
This study, conducted by researchers from IIT Delhi, Delhi Technological University, and IIT Kharagpur, tackles this very question by examining the ability of LLMs to understand and articulate the ‘Little Traditions’ of Indian society. These traditions encompass localized cultural practices, such as caste, kinship, marriage, and religion—elements that make up the fabric of India's rich and diverse cultural landscape. By analyzing how well LLMs recognize and respond to these nuanced traditions, the authors aim to uncover the extent to which AI systems can accommodate the complexities of localized cultures, often overshadowed by global or dominant cultural norms.
Understanding the Core Concepts:
-
Little Traditions vs. Great Traditions:
In the world of cultural anthropology, the distinction between Great and Little Traditions helps us understand how different layers of culture interact. Great Traditions represent the broad, elite, and often universalized cultural practices, while Little Traditions are the localized practices, deeply embedded in the everyday life of specific communities. This study uses these concepts to explore how well LLMs can recognize and respond to the diversity of Indian subcultures. -
Localized Cultural Practices:
India is home to a wide variety of localized cultural practices that can be seen in festivals, kinship norms, caste systems, and regional languages. These practices are often not widely represented in global discourse but are essential in shaping the social and cultural identity of the people who follow them.
The Challenge Addressed by the Research:
The core issue that this study addresses is the cultural bias inherent in many AI systems, particularly LLMs. These models are often trained on vast datasets that predominantly represent dominant cultures, leaving localized or minority cultures underrepresented. This can result in AI systems that fail to understand, or even misrepresent, the cultural nuances of these communities.
-
Current Limitations:
LLMs, despite their impressive language capabilities, tend to struggle with context-specific scenarios that require a deep understanding of local traditions. While they may perform well in generalized contexts, when it comes to discussing specific cultural practices like caste-based marriage rituals or the significance of regional festivals, LLMs often falter in providing nuanced or accurate information. -
Real-World Implications:
A lack of cultural sensitivity in AI systems can lead to inappropriate or offensive outputs, which can have severe consequences, particularly in sensitive areas like religion and social customs. This issue becomes even more pronounced in countries like India, where cultural practices vary dramatically across regions and communities.
A New Approach:
To assess how well LLMs perform in this area, the authors devised a novel methodology using case studies based on localized cultural practices within India. The study focused on four key aspects of Indian society: caste, kinship, marriage, and religion.
-
Innovative Solution:
The researchers used a combination of In-Context Learning (ICL) and zero-shot learning to test how well the LLMs could handle case studies that required understanding of specific cultural contexts. The LLMs were presented with instructions that asked them to choose between two options: one representing the dominant cultural view and the other representing a Little Tradition. They were also required to provide justifications for their choices. -
How It Works:
The models were tested using five popular LLMs: GPT-4, Llama-3.3-70b, Mixtral-8x7b-32768, and Gemini-1.5-flash. The prompts used were carefully crafted to probe the models' understanding of localized traditions and their ability to distinguish between Great and Little Traditions. For example, the models were asked to choose between the pan-Indian view of a religious festival and a localized variant of the same festival celebrated only in specific regions. -
Key Findings:
The study found that while LLMs were able to articulate cultural nuances, they often struggled to apply this understanding in real-world scenarios. This suggests that while LLMs are capable of generating culturally relevant responses, their practical understanding of local traditions is still lacking, particularly when nuanced context or regional diversity is involved.
Implications for the Field:
-
Practical Applications:
The ability of LLMs to understand and respect cultural diversity is critical for their deployment in various sectors, including education, healthcare, and customer service. For example, an AI-driven educational tool in India needs to be sensitive to regional languages, cultural practices, and social norms. Similarly, AI applications in healthcare should be able to understand the cultural context of patient care, which varies widely across India. -
Future Research:
This study paves the way for future research into enhancing LLMs' cultural sensitivity. Further studies could focus on incorporating regional language prompts or training models with more diverse datasets that include a broader representation of local cultures and traditions. There is also potential for AI developers to create models that specifically address cultural nuances in certain geographical or social contexts.
Conclusion: A Step Forward in AI and Cultural Sensitivity
This research represents an important step in evaluating how well AI systems, particularly LLMs, can engage with and understand cultural diversity. While current models show promise, there is still much work to be done in making AI systems more culturally aware and sensitive, especially when it comes to underrepresented traditions.
-
Summary:
By evaluating LLMs' understanding of Indian subcultures, the authors highlight the need for greater cultural inclusion in AI systems. Their findings underscore the importance of ensuring that AI models respect and accurately represent the full spectrum of cultural practices, from the Great Traditions to the Little Traditions. -
Broader Impact:
As AI becomes more integrated into society, its ability to understand and respect cultural diversity will play a crucial role in shaping its acceptance and trustworthiness. This research is a timely reminder of the need for continued efforts in making AI systems not only intelligent but also culturally intelligent.
Final Thoughts:
The challenge of embedding cultural diversity into AI systems is not just a technical issue—it is a moral and social one. As AI continues to shape our future, we must ensure that it is built to reflect the richness of the world’s cultures, acknowledging and respecting the many different ways in which people live, believe, and practice. The journey toward creating culturally aware AI is just beginning, and studies like this are crucial in guiding the way forward.
What are your thoughts on the potential for AI to embrace localized cultures? How can we ensure that AI systems are both technologically advanced and culturally respectful?
What's Your Reaction?
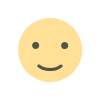
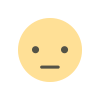
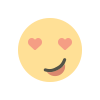
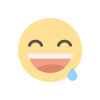
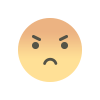
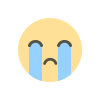
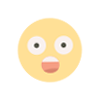